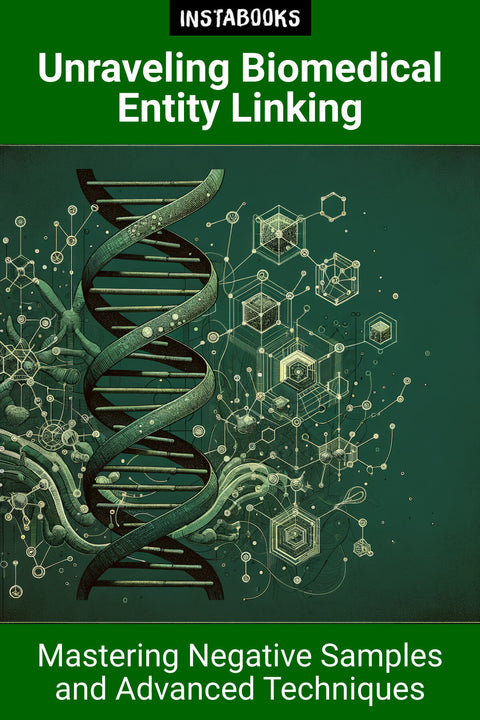
Instabooks AI (AI Author)
Unraveling Biomedical Entity Linking
Mastering Negative Samples and Advanced Techniques
Premium AI Book (PDF/ePub) - 200+ pages
Introduction to Biomedical Entity Linking
In the evolving field of biomedical informatics, Biomedical Entity Linking (BioEL) stands out as a pivotal process. This method involves mapping entities mentioned in biomedical texts to standardized ontology or knowledge bases like the Unified Medical Language System (UMLS) or Medical Subject Headings (MeSH). Such a task is indispensable for tasks such as relation extraction, information retrieval, and knowledge base construction. Our book, "Unraveling Biomedical Entity Linking," offers a comprehensive exploration of this crucial domain, equipping readers with a robust understanding of its mechanisms and applications.
Exploring Traditional Generative Models
Traditional generative models, like GENRE, have paved the way for BioEL by utilizing sequence-to-sequence models to generate candidates, subsequently re-ranking them through a classifier. However, their limitations become apparent when dealing with complex entity mentions and incorporating effective context. This book examines both their strengths and shortcomings, providing valuable insights into the development of more refined approaches.
The Role of Negative Samples in Enhancing Models
An often overlooked yet critical component in BioEL models is the inclusion of negative samples. Entities that appear similar but bear different meanings can significantly improve model accuracy. This section emphasizes why traditional models falter by usually relying solely on positive samples, leading to improper disambiguation. The book delves into methodologies for effectively integrating hard negative samples into generative models, allowing for more precise entity disambiguation.
Synonyms-Aware Fine-Tuning and Handling Complex Mentions
An innovative approach explored in this work is "Synonyms-Aware Fine-Tuning," which enriches generative models by infusing them with synonyms and definitions derived from knowledge bases. This chapter details how such techniques allow models to recognize and link differently named yet related entities, showcasing the enhanced proficiency in managing synonyms and context-dependent entities. Further, we investigate large language models' role in simplifying multi-token complex entity mentions, aiding in their effective normalization and linking.
Advancements Through Metric Learning-Based Methods
The latest developments in metric learning-based methods treat entities and their mentions holistically, irrespective of training set mentions quantity. Our book explains how these methods enhance a model’s capacity to capture intricate relationships between entities and their context, thus elevating the overall accuracy of linking efforts. Highlighted challenges include linking genes and proteins with current approaches, while future directions propose developing more advanced models that incorporate negative samples and employ synonyms-aware fine-tuning alongside potential multimodal ideas.
This book is an essential resource for researchers, data scientists, and students seeking to delve into the nuances of biomedical entity linking, offering pathways to refine models and methodologies in meaningful ways.
Table of Contents
1. Foundations of Biomedical Entity Linking- The Evolution of BioEL Models
- Standard Ontologies and Knowledge Bases
- Challenges in Entity Normalization
2. Traditional Generative Models
- Sequence-to-Sequence Approaches
- Context Incorporation Techniques
- Limitations and Gaps
3. The Impact of Negative Samples
- Understanding Hard Negatives
- Training with Diverse Samples
- Disambiguation Challenges
4. Introducing Synonyms-Aware Fine-Tuning
- Integrating Synonyms from Knowledge Bases
- Fine-Tuning Techniques
- Performance Implications
5. Handling Complex Entity Mentions
- Preprocessing Techniques
- Large Language Models in BioEL
- Simplification Strategies for Mentions
6. Metric Learning-Based Methods
- Holistic Entity Learning
- Capturing Nuanced Relationships
- Comparison with Traditional Methods
7. Linking Genes and Proteins
- Current Model Limitations
- Innovative Solutions
- Future Directions in Gene Linking
8. Incorporating Context in Linking Decisions
- Contextual Cues and Model Adaptation
- Challenges with Context Dependency
- Case Studies and Insights
9. Current Challenges in BioEL
- Complexity in Entity Linking
- Balancing Precision and Recall
- Common Pitfalls and Errors
10. Future Directions for Entity Linking Models
- Advances in Multimodal Fusion
- Enhancing Model Robustness
- Integration of Emerging Technologies
11. Recent Papers and Theoretical Insights
- Synonyms and Definitions in Model Training
- Evaluations of State-of-the-Art Models
- Exploration of New Metrics and Benchmarks
12. Tools, Benchmarks, and Resources
- Open-Source Toolkits
- Benchmark Datasets and Leaderboards
- Community Contributions and Collaborations
Target Audience
This book is written for researchers, data scientists, and students interested in advancing their knowledge and techniques in biomedical entity linking.
Key Takeaways
- Understand the foundational concepts and challenges of Biomedical Entity Linking (BioEL).
- Explore the importance of negative samples in enhancing model accuracy.
- Learn about synonyms-aware fine-tuning and its impact on entity linking.
- Discover recent advancements in metric learning-based methods.
- Gain insights into handling complex entity mentions with large language models.
- Equip yourself with knowledge on current challenges and future directions in BioEL.
How This Book Was Generated
This book is the result of our advanced AI text generator, meticulously crafted to deliver not just information but meaningful insights. By leveraging our AI book generator, cutting-edge models, and real-time research, we ensure each page reflects the most current and reliable knowledge. Our AI processes vast data with unmatched precision, producing over 200 pages of coherent, authoritative content. This isn’t just a collection of facts—it’s a thoughtfully crafted narrative, shaped by our technology, that engages the mind and resonates with the reader, offering a deep, trustworthy exploration of the subject.
Satisfaction Guaranteed: Try It Risk-Free
We invite you to try it out for yourself, backed by our no-questions-asked money-back guarantee. If you're not completely satisfied, we'll refund your purchase—no strings attached.