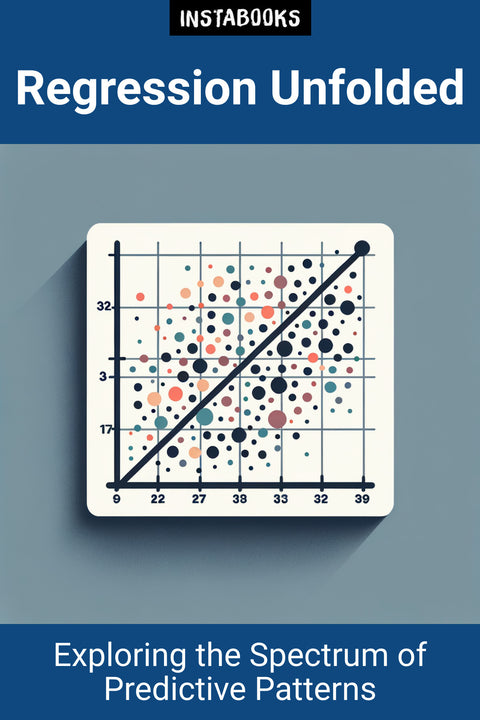
Instabooks (AI Author)
Regression Unfolded
Exploring the Spectrum of Predictive Patterns
Premium AI Book (PDF/ePub) - 200+ pages
Table of Contents
1. The Essence of Regression- Understanding Data Relationships
- History and Evolution of Regression
- Major Types and Uses
2. Linear Regression in Depth
- Fundamentals of Linear Relationships
- Assumptions and Properties
- Practical Applications and Case Studies
3. Diving Into Logistic Regression
- From Linear to Logistic: The Transition
- Binary Outcomes and Odds Ratio
- Case Studies: Medical and Social Sciences
4. Polynomial Regression
- Beyond Linearity: Capturing Curvature
- Determining the Degree of Polynomials
- Polynomial Regression in the Wild
5. Ridge and Lasso Regression
- Tackling Multicollinearity
- Balance Between Fit and Complexity
- Ridge vs. Lasso: A Comparative Analysis
6. Elastic Net Regression
- Combining L1 and L2 Penalties
- Parameters Selection and Optimization
- Application in High-Dimensional Data
7. Quantile Regression
- Percentiles in Regression Analysis
- Advantages Over Ordinary Least Squares
- Applications in Economics and Finance
8. Generalized Linear Models
- Expanding Beyond Normality
- Link Functions and Exponential Families
- Model Selection and Evaluation
9. Non-Linear and Non-Parametric Regression
- When Linearity Fails
- Kernel Methods and Splines
- Case Studies: Complex Data Patterns
10. Survival and Hazard Regression
- Time-to-Event Data Analysis
- Cox Proportional-Hazards Model
- Predictive Modeling in Healthcare
11. Mixed Models and Hierarchical Data
- Random Effects and Nested Structures
- Application in Longitudinal Studies
- Software for Mixed Model Analysis
12. Machine Learning and Regression
- Regression Trees and Forests
- Support Vector Regression
- Neural Networks and Deep Learning
How This Book Was Generated
This book is the result of our advanced AI text generator, meticulously crafted to deliver not just information but meaningful insights. By leveraging our AI book generator, cutting-edge models, and real-time research, we ensure each page reflects the most current and reliable knowledge. Our AI processes vast data with unmatched precision, producing over 200 pages of coherent, authoritative content. This isn’t just a collection of facts—it’s a thoughtfully crafted narrative, shaped by our technology, that engages the mind and resonates with the reader, offering a deep, trustworthy exploration of the subject.
Satisfaction Guaranteed: Try It Risk-Free
We invite you to try it out for yourself, backed by our no-questions-asked money-back guarantee. If you're not completely satisfied, we'll refund your purchase—no strings attached.